The ongoing conversation linked to AI and its potential uses has spanned industries in a very short amount of time. From the release of ChatGPT and schools’ subsequent concerns about the technology to AI’s recent usage to mimic internationally recognized voices of celebrities such as Drake and the Weeknd, artificial intelligence (AI) has been a topic on many of our minds recently.
The healthcare industry stands as no exception, especially as the technology continues to develop and improve, maximizing its potential applications for providers.
Keith A. Hovan is a healthcare CEO, strategist, and clinician who has been very interested in developments within AI technology and the value that it can bring to healthcare settings.
Here, Mr. Hovan addresses a variety of considerations for healthcare providers and leaders as the industry continues to navigate the technology and find innovative ways to leverage its usage.
Establishing the Meaning of AI in a Healthcare Context
Before diving into the considerations of AI technology, it is important to first explore what AI means in a healthcare context.
AI, or machine learning, refers to computer programs supplied with incredible amounts of data through text, images, video, sound, numbers, or other encoded sources of information This data is analyzed, allowing the AI to draw inferences based on patterns found within the data.
In healthcare settings, oncologists could include millions of pictures of skin ranging from normal to abnormal onto a computer. With this data, the computer can utilize AI to find patterns making it possible to identify abnormalities such as cancerous skin lesions through matches.
A benefit of AI’s potential uses for healthcare delivery is that its ability to collect and compare data empowers it to save a lot of time. The value here is that it could free up administrative time for healthcare professionals who could, in turn, spend longer providing care for their patients.
What’s more is that this is just the beginning— there are so many potential uses for this technology and we are only scratching the surface.
Interpretation is Key
It is important to remember that, despite AI’s strengths, it does have a few inherent weaknesses. AI is an efficient tool to search for patterns and has the ability to log and arrange coherent data, however, the technology is limited by its lack of ability to synthesize this information, further evaluate its quality, form a constructive argument, or make decisions with data.
This means that machine learning is not going to serve as a complete fix for all problems on the user side and there is always the capacity for AI to create potential new issues along the way. Given the stakes in healthcare environments, those in charge have many things to consider as they work to evaluate whether or not AI can be used in certain situations.
AI technologies fall into two categories, general purpose and generative.
Generative AI is a type of artificial intelligence that creates images or text from a series of previous examples. This type of AI is used to create an output that is based on predictive analysis by being trained on copious amounts of data.
General purpose AI is artificial intelligence that is not created for a specific purpose or application. This means that it does not perform at the same level of generative AI. ChatGPT is an example, being that it is optimized for dialogue but is not as effective for complex computations and the like.
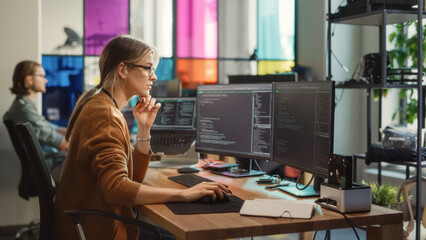
What’s true of both types of AI at this stage in its development is that they require human oversight and intervention to properly benefit most work — healthcare settings included. AI’s role, therefore, is likely to be more supplemental rather than a replacement for current processes or professionals. AI’s most effective use is in situations where it can be fed a lot of data and check for patterns among the information that is received.
The ability to be given data and search for patterns opens up several incredible uses for AI technology in our current landscape. For example, AI technology can be effectively leveraged to better promote patient safety through flagging risk factors, potential complications, and issues that could promote early intervention. These scenarios could improve health outcomes for patients suffering from a variety of conditions.
Keith A. Hovan also finds that machine learning can also play a role in determining the course of cancer treatment for patients. Through amassing data on location, types of malignancy, duration, and several other factors, combined with information on patients with similar conditions, recommendations for effective treatment options that promote better health outcomes could turn what sounds like science-fiction into our reality.
Both examples will necessitate human interference to interpret flagged data, process it in context, and evaluate the findings. For this reason, Keith currently views AI as a great collaborator but certainly not a source of information that can be trusted and utilized without question. There are biases and the potential for accuracy related risks inherent to AI technology, but AI is a clear starting point that can save a lot of time and energy if used well.
Data Relevance in AI Usage
On its own, AI is not intelligent. AI merely mimics human intelligence through the data that it collects. With this in mind, AI needs to be checked diligently for truly effective results. Our responsibility when leveraging the technology is to ensure that AI’s output is not biased by the information that it is fed.
With healthcare delivery as an example, if data that is available for a certain condition is largely based on America Caucasian males, output from the AI won’t be as widely helpful in a larger context. Instead, its use could be quite dangerous for patients who do not fit that profile but are given feedback or advice based on AI’s output.
Something to consider is the fact machine learning’s output is only as good as the data that it is being supplied. AI is probabilistic and will make predictions based on data and prompts as well as their quality.
ChatGPT can be used as an example here. If you ask ChatGPT imprecise or silly questions, you will get imprecise or silly answers as an output. This also applies to generative forms of AI being leveraged in healthcare settings. Human interference can be effective at gatekeeping to ensure that only relevant data is inputted and that providers only follow up on the best applications of AI’s recommendations.
Cultural Context’s Impact on AI’s Output
Cultural context is extremely important in the AI discussion. After all, each section of the world has its own regional and cultural context, ethical principles, considerations, moral structures, religious components, etc. working in tandem. This makes them unique.
At the time of this article’s writing, AI is not yet able to account for the millions of cultural differences and nuances across the board. This ensures that there will be a lot of variance in AI’s effectiveness depending on where the technology is being used.
Language models in AI usage are provided with a lot of data in the form of text inputs wherein they are in a specific language. A challenge here is that the use of a language alongside its norms, be they ethnic, religious, or national, can unintentionally create bias should you try to apply them to non-related groups or populations.
For example, most language models are built in English which already creates an immediate issue with bias if the user speaks a character based language such as Japanese instead. Language models can also “hallucinate” meaning that they can create their own interpretations of inputs based on false, unvetted data or misunderstood patterns within language.
Because English is the most digitized language, there will be social biases in language models inherently. With this, there is the potential for biases against protected classes in our communities such as people of color, women, or people who identify as LGBTQAI+.
In healthcare settings, professionals have worked very diligently for equity in terms of delivery and recognizing the challenges to providing quality care for diverse populations of people. Healthcare leaders have also made strides to ensure care delivered to patients adheres to guidelines and builds towards high quality care.
Simply put, it has been a real journey creating and maintaining an ethical landscape for healthcare. Keith A. Hovan along with many others have to wonder if introducing AI into the fold of healthcare processes can make things worse rather than better if it is not properly vetted.
Regulation and Compliance Issues for AI
Safeguarding against cultural biases in AI is essential for healthcare leaders, institutions, and government officials as they continue to wrestle with the technology.
How high level will input data moderation need to be? Will we easily be able to differentiate AI-generated outcomes from one’s performed by humans? How do we incorporate elements of human interpretation or common sense without sacrificing sought after efficiencies? These are important questions to ask as we think about regulation and compliance.
AI left to its own devices could reinforce inequities and even create them if we are not careful. Currently, sixteen U.S. states have some active form of AI regulation, however, effective regulation is another thing entirely. In its current state, AI regulation is not able to adapt to the rapid pace at which machine learning is developing. Potential compliance issues present a hurdle for any highly regulated industries.
Another factor in the greater AI regulation and compliance discussion is HIPPA as compliance issues have hindered AI usage in certain applications as well as the amassing and input of protected data. For AI to have more influence on the healthcare landscape, both regulatory and compliance standards need to keep pace with AI’s advancement while working to protect vulnerable members of our communities.
Conclusion
As AI technology continues to advance and have a larger presence in our lives, it will further influence how we interact with and experience our world. Keith A. Hovan upholds that there will likely be evolving social and ethical issues that impact healthcare and various other industries along the way.
Still, Keith A. Hovan and other healthcare professionals remain committed to the guiding philosophy of Hippocrates, “do no harm”. To this point, it is absolutely crucial that professionals, leaders, and institutions as a whole work to ensure that they cause no harm to the people that they serve as AI technologies advance and usage becomes more widespread over time.
Properly covering the bases will mean that we do not hide from the fact that AI, in its current state, has a set of significant limitations. Much like Tesla’s Autopilot, AI is most at home as an “electronic co-pilot” of sorts, harnessing the potential to make human providers more effective in their roles. It should not be heavily relied on — at least not yet.
Sources
Irene Solaiman, Hugging Face
Gidian Lichfield, OpenAI
Seth Dobrin, Responsible AI Institute
Tom Simonite, Wired
Lama Nazer
Suchi Saria, AI Professor, Johns Hopkins, CEO, Bayesian Health
Dr. Azra Bihorac, Dean of Research, UFCM
Dr. Tiffany Kung, Researcher, AnsibleHealth